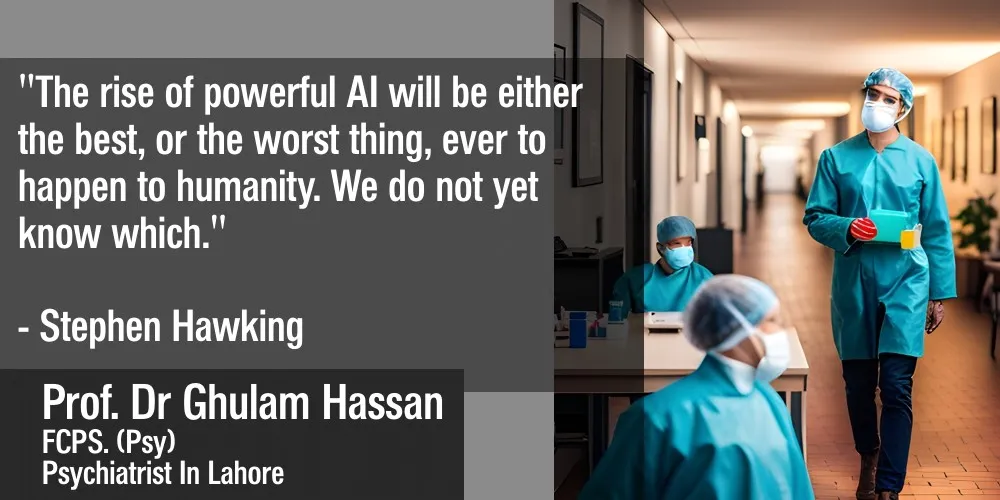
Understanding Generative AI
In a world where technology seems to leapfrog boundaries with each passing day, generative artificial intelligence (AI) emerges as a remarkable standout. At its core, generative AI possesses an extraordinary ability—the power to create. It’s not just about computations; it’s about creativity. Generative AI, a subset of artificial intelligence, doesn’t merely process data; it generates new content based on patterns it learns from existing information.
In the context of drug discovery, this AI marvel acts as a digital alchemist. It delves into the intricate world of molecules, interactions, and compounds, deciphering the language of chemistry. Generative AI becomes a virtual laboratory assistant, combining elements and structures to craft novel molecular possibilities that scientists might not have encountered before.
“The advance of technology is based on making it fit in so that you don’t really even notice it, so it’s part of everyday life.” – Bill Gates
But its applications extend beyond the realm of pharmaceuticals. Generative AI’s innovative prowess infiltrates various fields, from art and music to literature and design. Picture an AI composer crafting symphonies or an AI author drafting eloquent prose. It’s the technological equivalent of breathing life into the canvas of creativity, redefining the possibilities of what human imagination combined with machine intelligence can achieve.
Challenges in Traditional Drug Discovery
The landscape of traditional drug discovery is marked by a series of formidable challenges that have long impeded the journey toward groundbreaking medications. The conventional approach involves an arduous and often painstaking process of screening vast libraries of compounds in search of that elusive gem—a potential drug candidate. This task, akin to finding a needle in a haystack, demands monumental resources, time, and effort.
“Artificial intelligence, deep learning, machine learning—whatever you’re doing if you don’t understand it—learn it. Because otherwise, you’re going to be a dinosaur within 3 years.” – Mark Cuban
Moreover, the process is riddled with uncertainties. The identification of a compound with therapeutic potential is like uncovering a hidden treasure, obscured by a myriad of false leads and ambiguous outcomes. The trial-and-error nature of traditional methods, coupled with the extensive labor and financial investment required, paints a portrait of an industry in need of transformation.
The Need for Innovative Approaches
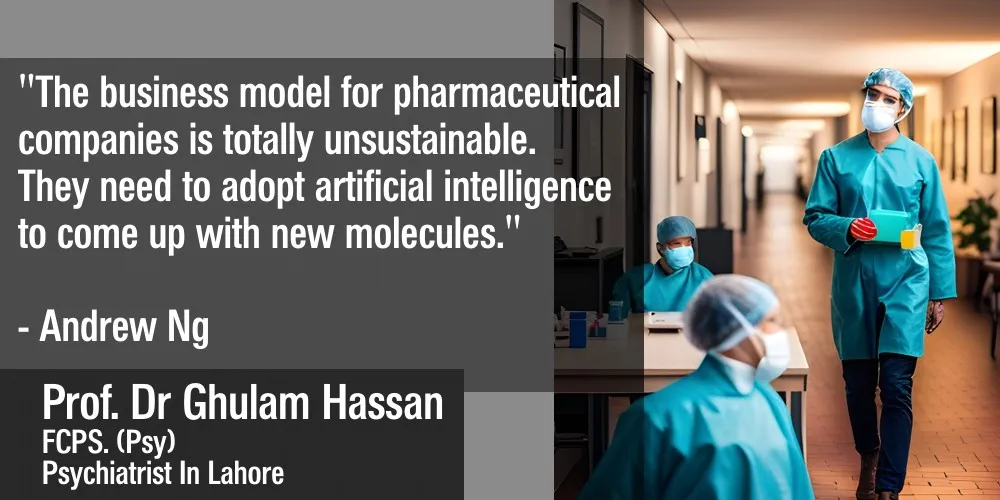
As the demand for effective medications grows and the urgency to combat complex diseases intensifies, a paradigm shift becomes imperative. Innovative approaches that can untangle the complexities of drug discovery are not just desirable; they’re essential. The traditional route, though undeniably valuable, often struggles to keep pace with the rapid advancement of medical knowledge.
Enter generative AI, the beacon of innovation that offers a refreshing perspective on drug discovery. By infusing the process with unparalleled speed, efficiency, and a touch of technological wizardry, generative AI holds the promise of unlocking solutions that have long eluded traditional methods. Its ability to navigate the labyrinth of molecular interactions with precision and creativity injects hope into a landscape hungry for progress. The need for innovative acceleration is no longer a whisper—it’s a resounding call to action.
Generative AI in Molecule Design
In the intricate world of drug discovery, where every molecule holds the potential to change lives, the process of designing novel drug molecules demands precision and ingenuity. This is where generative AI steps onto the stage with its virtual toolkit of innovation.
Generative AI, akin to a master architect, analyzes vast databases of existing molecules, drawing from a repository of chemical structures and interactions. Armed with this knowledge, it takes on the role of a molecular composer, crafting new possibilities by intelligently combining fragments and elements. These generated molecules, while born from algorithms, possess the potential to be real-world drug candidates.
Speed and Efficiency Unleashed
Perhaps one of the most striking features of generative AI in molecule design is the speed and efficiency it injects into a process that traditionally unfurls at a slower pace. While human researchers would painstakingly sift through compounds, generative AI works at a pace that can only be described as lightning-fast. It explores a multitude of permutations and combinations in mere seconds, generating a spectrum of molecules that hold promise.
This acceleration is nothing short of revolutionary. What might have taken months, or even years, can now be accomplished in a fraction of the time. The significance of this leap cannot be overstated, especially in a field where time is of the essence and lives hang in the balance. The emergence of generative AI in molecule design isn’t just a technological upgrade—it’s a transformative force that’s propelling drug discovery into a new era of possibilities.
Enhancing Compound Library Creation
In the intricate dance of drug discovery, the quality and diversity of the compound library can make all the difference. A rich, varied library increases the chances of discovering that elusive molecule with therapeutic potential. Generative AI emerges as a virtuoso conductor in this symphony of molecules, enhancing the creation of compound libraries like never before.
Generative AI, armed with its keen understanding of chemical interactions and structures, takes the concept of diversity to new heights. It explores uncharted territories of chemical space, suggesting compounds that might have been overlooked using traditional methods. It’s as if generative AI is a curator of a gallery of molecules, introducing art pieces that challenge conventions and broaden perspectives.
Impact on Identifying Potential Drug Candidates
The ramifications of generative AI’s role in compound library creation extend far beyond its creative suggestions. The molecules it proposes aren’t merely abstract entities; they hold the potential to be the missing puzzle pieces in the grand picture of drug discovery. The diverse library generated by AI serves as a playground for scientists, a treasure trove of novel compounds to be examined and evaluated.
As researchers navigate through this virtual cornucopia, they unearth potential drug candidates that might have otherwise remained hidden. The diverse molecular tapestry laid out by generative AI enables a more comprehensive exploration of possibilities, increasing the likelihood of identifying molecules that possess the sought-after combination of efficacy and safety.
Generative AI doesn’t just enhance compound library creation—it revolutionizes it. Its impact ripples through the entire drug discovery process, offering a richer palette of options and reshaping how potential drug candidates are unearthed and evaluated.
Predicting Drug-Target Interactions
The intricate dance between drugs and biological targets forms the core of effective drug development. Understanding how a drug molecule interacts with its intended target is like deciphering a secret language—a language that generative AI is becoming increasingly fluent in.
Generative AI approaches the task of predicting drug-target interactions with a blend of analytical prowess and machine learning finesse. It analyzes vast datasets containing information about drug molecules, their structures, and the biological targets they aim to affect. By recognizing patterns and relationships within this data, generative AI establishes a blueprint for potential interactions.
Emphasizing Affinity and Efficacy
Within this realm of drug-target interactions, the concept of affinity takes center stage. Affinity refers to the strength of the binding between a drug molecule and its biological target. The higher the affinity, the more effectively the drug can engage with the target and produce the desired effects.
Generative AI’s role in predicting drug-target interactions is instrumental in identifying compounds with high affinity. By analyzing structural details, chemical properties, and the complex dynamics of interactions, AI algorithms unearth potential drug candidates that exhibit strong binding capabilities. This predictive power enables researchers to focus their attention on compounds that are more likely to be efficacious, accelerating the process of selecting promising candidates for further exploration.
In a world where precision matters, generative AI stands as a guide that navigates the labyrinth of molecular interactions, leading researchers toward compounds that hold the promise of unlocking new therapies and transforming lives.
Accelerating Preclinical Research
The journey from a potential drug candidate to a life-saving medication is marked by multiple stages of evaluation and testing. The preclinical phase, where compounds are rigorously examined for efficacy and safety, is a critical juncture in this path. Generative AI emerges as a formidable ally in this phase, revolutionizing the process by expediting the identification of viable candidates.
Generative AI doesn’t merely assist; it propels preclinical research forward with an unparalleled velocity. By sifting through vast pools of data, AI algorithms recognize patterns that elude human observation. These patterns, often indicative of a compound’s potential, guide the AI’s suggestions toward molecules worthy of consideration.
Reducing Costs and Time
The impact of generative AI on preclinical research is not only measured in its capacity to suggest promising candidates; it’s also a champion of efficiency. Traditional methods are often marked by lengthy trial-and-error cycles, where compounds are meticulously tested, and results are painstakingly evaluated. Generative AI introduces a touch of rapidity into this process, narrowing down the pool of candidates by focusing on those with the highest likelihood of success.
This acceleration isn’t just a matter of speed—it’s a matter of resources. As generative AI refines the selection process, it paves the way for cost savings that can be directed toward further research and development. Moreover, time is a precious commodity in drug discovery, especially when lives hang in the balance. By reducing the time spent in preclinical research, generative AI aligns with the urgent needs of patients and researchers alike, accelerating the journey from hypothesis to hope.
Combating Rare Diseases
In the vast landscape of medical research, some battles are waged against adversaries that lurk in the shadows—rare and orphan diseases, often neglected due to their infrequency. Generative AI emerges as a potent weapon in this fight, offering a glimmer of hope for those who have long awaited solutions.
Unlocking Potential with Limited Data
Rare diseases present a unique challenge: a scarcity of data. Traditional research methods often rely on extensive datasets to derive meaningful insights. However, generative AI thrives in environments where data is limited. It possesses the remarkable ability to uncover hidden patterns and relationships even in the midst of scarcity.
A Beacon of Innovation
Generative AI’s role in combating rare diseases is nothing short of revolutionary. It embraces the challenge of limited data and transforms it into an opportunity for innovation. By analyzing what little data is available, AI algorithms generate solutions that are both ingenious and unexpected. It’s like solving a puzzle with missing pieces—AI fills in the gaps with creative suggestions that lead researchers down new paths of exploration.
This approach breathes life into the concept of personalized medicine for rare diseases. The uniqueness of each case demands solutions that are equally unique. Generative AI, with its ability to craft tailored molecular possibilities, stands as a beacon of innovation that illuminates the way forward in the battle against diseases that have long eluded traditional methods.
Shattering Boundaries
Generative AI doesn’t just combat rare diseases; it shatters the boundaries that confine our thinking. It’s a reminder that solutions can emerge from unexpected places, that innovation can flourish even in the face of adversity. In the world of rare diseases, where hope has often felt distant, generative AI extends an invitation to explore uncharted territories, to dare to dream of possibilities that were once considered unattainable.
Addressing Safety and Toxicity
In the intricate dance of drug discovery, efficacy is only one part of the equation. Safety and toxicity play an equally crucial role in determining whether a potential drug candidate will navigate the journey from the laboratory to the medicine cabinet. Generative AI emerges as a vigilant guardian, equipped with the power to predict potential safety concerns and toxicity issues that could arise during drug development.
Predictive Insights
Generative AI’s ability to predict safety and toxicity issues is rooted in its keen understanding of molecular structures and interactions. By analyzing data on existing compounds and their interactions with biological systems, AI algorithms discern patterns that can indicate potential risks. These patterns often manifest as structural elements associated with toxicity or interactions that could trigger adverse effects.
Minimizing Risks in Drug Development
The role of generative AI in predicting safety and toxicity issues is not merely about identifying problems—it’s about preventing them. By highlighting potential concerns early in the drug development process, AI provides researchers with a roadmap to navigate the path ahead more cautiously.
This proactive approach to risk assessment is a game-changer. Traditional methods often rely on trial and error, with safety concerns sometimes emerging late in the development process, leading to setbacks and even failures. Generative AI’s role in minimizing risks is akin to a navigator steering a ship away from treacherous waters. It ensures that potential pitfalls are anticipated and mitigated, safeguarding both patients and the progress of drug development.
A Safety Net of Innovation
Generative AI’s predictive prowess doesn’t just minimize risks—it enhances the entire drug development journey. By addressing safety and toxicity concerns at the outset, AI empowers researchers to make informed decisions, streamlining the process and reducing the likelihood of costly setbacks. In a world where safety is paramount, generative AI stands as a safety net of innovation, supporting the pursuit of effective treatments while ensuring patient well-being remains at the forefront.
Human-AI Collaboration
The narrative of technological advancement has often been framed as a clash between humans and machines—a tale of man versus the machine. However, in the realm of drug discovery, a new chapter is being written—one that celebrates the harmony of human-AI collaboration. This partnership isn’t about humans being replaced by algorithms; it’s about the fusion of human intellect and AI precision to unlock a new era of innovation.
A Symbiotic Relationship
At its core, human-AI collaboration in drug discovery is a symbiotic relationship that capitalizes on the strengths of both parties. Humans bring creativity, intuition, and an understanding of the broader context to the table. AI algorithms, on the other hand, wield the power of rapid data analysis, pattern recognition, and prediction. It’s a marriage of minds—one that enhances the capabilities of each.
The Dance of Complementary Skills
Imagine a researcher armed with a question—a hypothesis that requires validation through data analysis. Here’s where AI algorithms step in, crunching numbers at lightning speed to uncover patterns that would take humans significantly longer to identify. The researcher, in turn, interprets these insights in the context of the broader scientific landscape, adding nuance and depth to the analysis.
This dance of complementary skills is a hallmark of human-AI collaboration. It’s a partnership that transcends traditional boundaries, transforming drug discovery into a dynamic, iterative process. Humans pose questions, AI algorithms provide answers, and together they fine-tune the trajectory of research.
Shaping a New Frontier
Human-AI collaboration isn’t a replacement for human researchers; it’s an invitation to redefine the boundaries of innovation. The insights generated by AI algorithms are not conclusions; they’re starting points. They initiate discussions, spark hypotheses, and serve as beacons guiding human researchers toward uncharted territories of exploration.
In a world where the complexities of drug discovery continue to evolve, human-AI collaboration isn’t just a fleeting trend—it’s a new frontier that’s reshaping the landscape of medical research. It’s a declaration that the future belongs to those who dare to bridge the gap between human ingenuity and machine intelligence, forging a path toward solutions that were once beyond imagination.
Ethical Considerations
In the awe-inspiring journey of integrating generative AI into drug discovery, the promise of innovation and acceleration is accompanied by a responsibility that cannot be ignored—the ethical implications of harnessing artificial intelligence for scientific progress. As we navigate the uncharted waters of this technological revolution, we must carefully examine the ethical compass guiding our actions.
The Balancing Act
Generative AI’s ability to predict, design, and innovate brings with it a dual nature—a gift of progress and a challenge of responsibility. The rapidity with which AI algorithms generate insights can lead to a temptation to overlook the meticulous validation that science demands. There’s a delicate balance to strike—between the eagerness to advance and the need for rigorous scrutiny.
The Call for Transparency
Transparency stands as a cornerstone of responsible AI implementation. The decisions made by AI algorithms should be demystified, with the rationale behind their choices accessible to human researchers. The goal isn’t to replace human judgment with machine decision-making; it’s to enrich human judgment with AI-generated insights.
Safeguarding Patient Well-being
Ultimately, the pursuit of scientific advancement should always be aligned with the well-being of patients. Generative AI’s role in predicting drug safety and interactions is not just about molecules—it’s about individuals who place their trust in the medications that emerge from these discoveries. Ensuring patient safety is a responsibility that transcends innovation, one that demands ethical considerations take precedence.
Importance of Responsible AI Implementation
The importance of responsible AI implementation in drug discovery cannot be overstated. The road to progress must be paved with integrity, transparency, and a commitment to the welfare of humanity. Ethical considerations are not mere obstacles; they are the moral compass guiding us toward a future where innovation and integrity coexist harmoniously.
Responsible AI implementation doesn’t stifle progress—it enhances it. It elevates the potential of generative AI from a tool of discovery to a beacon of trust. It signifies a commitment to scientific advancement that’s rooted in principles and anchored in the shared values of the scientific community and society at large.
Future Prospects and Innovations
As the curtain rises on the present, the stage is set for generative AI to step into the spotlight of drug discovery. But this is not just a fleeting cameo; it’s a role with the potential to shape the entire narrative of medical research. The future prospects of generative AI in drug discovery are as promising as they are awe-inspiring, offering a glimpse into the untapped potential that lies ahead.
The Evolution of AI Algorithms
Generative AI algorithms are not static entities; they evolve and adapt with each iteration. As technology advances, we can expect AI algorithms to become more sophisticated, fine-tuned to the nuances of molecular interactions. This evolution is poised to catalyze an even greater accuracy in predictions, a refinement that could drastically reshape the landscape of drug development.
Personalized Medicine on the Horizon
The era of personalized medicine is inching closer, and generative AI is at the forefront of this revolution. The ability of AI algorithms to analyze individual patient data and tailor treatments accordingly is a tantalizing prospect. It’s a future where medications are as unique as the individuals they aim to help, a future that transcends the one-size-fits-all approach that has dominated medicine for decades.
The Rise of Multi-Modal AI
The fusion of multiple AI modalities—text, images, and data—heralds an era of comprehensive understanding. In drug discovery, this could mean AI algorithms that not only predict molecular interactions but also visualize them. Imagine AI-generated visual representations of chemical reactions and interactions, offering researchers insights that were once confined to the realm of imagination.
Emerging Trends and Ongoing Research
Amidst this whirlwind of innovation, numerous trends and ongoing research efforts are shaping the trajectory of generative AI in drug discovery. Researchers are delving into the intricacies of AI-generated compounds, exploring their viability and potential. The collaboration between academia, pharmaceutical companies, and AI pioneers is fostering a robust ecosystem that thrives on collective expertise.
Moreover, the field of explainable AI—a discipline that aims to decipher the decision-making processes of AI algorithms—is gaining momentum. This trend is critical in ensuring that the insights provided by AI are not just accepted but understood, adding a layer of transparency to the decision-making process.
The future of generative AI in drug discovery is a tapestry woven with threads of innovation, collaboration, and ethical consciousness. It’s a journey that’s poised to challenge conventions, transcend limitations, and uncover solutions that were once deemed impossible. As the curtain falls on the present, it rises on a future that beckons us to explore, to innovate, and to embrace the boundless potential of generative AI in the realm of medicine.
FAQs: Generative AI in Drug Discovery
Q1: What is generative AI in drug discovery?
Generative AI in drug discovery is the utilization of artificial intelligence algorithms to predict, design, and generate new drug molecules, facilitating the search for potential candidates with therapeutic properties.
Q2: How does generative AI work in drug discovery?
Generative AI analyzes existing data on molecules, their interactions, and structures, and then uses machine learning techniques to create new molecular possibilities by combining and modifying existing elements.
Q3: What are the benefits of using generative AI in drug discovery?
Generative AI accelerates the drug discovery process by rapidly suggesting novel compounds, potentially reducing time and costs associated with traditional methods.
Q4: Can generative AI design molecules that are safe and effective?
Generative AI can predict potential safety and toxicity concerns, enhancing the chances of identifying molecules with higher efficacy and safety profiles.
Q5: How does generative AI accelerate preclinical research?
Generative AI expedites preclinical research by suggesting viable candidates for testing, thereby reducing the time required to identify potential drug candidates.
Q6: Can generative AI be used for rare diseases?
Yes, generative AI’s ability to work with limited data makes it valuable in tackling rare and orphan diseases where data scarcity is a challenge.
Q7: How does generative AI predict drug-target interactions?
Generative AI predicts drug-target interactions by analyzing existing data on drug molecules and biological targets, recognizing patterns that indicate potential binding.
Q8: Is generative AI replacing human researchers in drug discovery?
No, generative AI complements human researchers by providing rapid insights, aiding decision-making, and accelerating the drug discovery process.
Q9: How does human-AI collaboration work in drug discovery?
Human-AI collaboration involves researchers posing questions and AI algorithms providing data-driven insights, which researchers then interpret in context to make informed decisions.
Q10: What are the ethical implications of using generative AI in drug discovery?
Ethical considerations involve ensuring transparency, accountability, and patient safety while harnessing AI’s potential in drug discovery.
Q11: Can generative AI help combat resistance in drug development?
Yes, generative AI can aid in designing molecules that tackle drug resistance by predicting interactions and optimizing properties for increased efficacy.
Q12: How does generative AI handle the complexity of molecular interactions?
Generative AI uses machine learning algorithms to understand complex interactions, learning from patterns in data to generate new molecular structures.
Q13: Is generative AI used only for small molecules or larger biomolecules as well?
Generative AI can be used for both small molecules and larger biomolecules, making it versatile in various aspects of drug discovery.
Q14: Can generative AI assist in repurposing existing drugs for new indications?
Yes, generative AI can explore existing drug libraries and suggest potential new uses for known compounds, aiding drug repurposing efforts.
Q15: Does generative AI replace experimental testing in drug discovery?
No, generative AI augments experimental testing by suggesting candidates for testing, optimizing resources and efficiency.
Q16: Can generative AI help in designing personalized treatments?
Yes, generative AI’s ability to analyze individual patient data makes it promising for designing personalized treatments based on patient profiles.
Q17: Are there any limitations to using generative AI in drug discovery?
Generative AI relies on the data it is trained on and may face challenges with novel situations or limited data availability.
Q18: How does generative AI contribute to innovative drug design?
Generative AI suggests novel molecular structures that researchers might not have considered, contributing to innovative approaches in drug design.
Q19: What are the implications of using generative AI in intellectual property rights?
The use of generative AI in drug discovery can raise questions about patenting and ownership of AI-generated compounds.
Q20: Can generative AI predict potential side effects of drugs?
Yes, generative AI can analyze molecular structures and predict potential side effects based on patterns learned from existing data.
Q21: How does generative AI impact the drug development timeline?
Generative AI accelerates the drug development timeline by suggesting candidates early in the process, potentially reducing time spent on trial and error.
Q22: Can generative AI help in drug formulation and delivery?
Yes, generative AI can assist in optimizing drug formulations and delivery mechanisms, enhancing drug efficacy and patient experience.
Q23: What role does generative AI play in reducing the cost of drug development?
Generative AI’s rapid suggestions and efficient candidate selection can reduce the costs associated with traditional trial and error methods.
Q24: Is generative AI accessible to all researchers?
The accessibility of generative AI varies, with some tools and platforms available to researchers while others may require specialized resources.
Q25: How does generative AI handle the complexity of biological systems?
Generative AI uses machine learning techniques to learn from complex biological data, enabling it to predict interactions and suggest potential candidates.
Q26: What is the role of generative AI in drug repurposing?
Generative AI can analyze existing drug libraries, identifying potential new uses for known compounds and accelerating drug repurposing efforts.
Q27: Can generative AI be used for target identification in drug discovery?
Yes, generative AI can aid in target identification by analyzing biological data and suggesting potential interactions and pathways.
Q28: How can generative AI improve the hit-to-lead optimization process?
Generative AI can suggest novel compounds during hit-to-lead optimization, increasing the chances of identifying successful leads for further development.
Q29: Are there regulatory challenges when using generative AI in drug discovery?
Yes, regulatory bodies may need to adapt to the use of AI-generated compounds and ensure safety and efficacy standards are met.
Q30: What is the long-term impact of generative AI on the pharmaceutical industry?
Generative AI has the potential to revolutionize drug discovery by accelerating the process, reducing costs, and paving the way for innovative treatments for various diseases.
1 thought on “Role of Generative AI in Drug Discovery – A Detailed Guide”